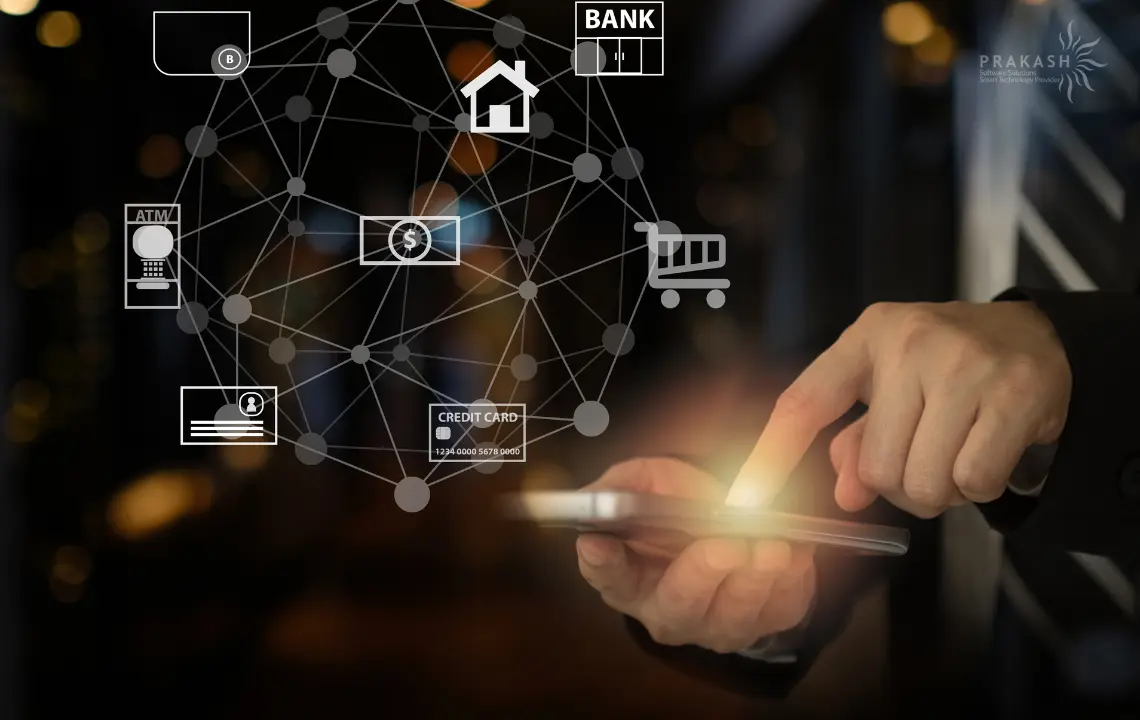
Comprehensive Guide to Generative AI’s Potential in Banking
Most of us are acquainted with “generative AI,” which creates various content forms like text, images, music, and synthetic data. The recent buzz surrounding generative AI is attributed to its user-friendly interfaces, swiftly producing high-quality text, images, and videos. Generative AI advances beyond traditional AI, allowing machines to generate content and interact with humans more authentically.
While AI clearly has a great deal of potential to revolutionize financial institutions’ operations, increase productivity, and promote innovation. It is important to keep in mind that its real value comes from enhancing rather than completely replacing human expertise. Financial services enhance operations and client experiences by integrating generative AI into workflows.
This blog explores generative AI’s impact on banking, uncovering envisioned benefits and significant implications.
McKinsey estimates generative AI could yield $200-340 billion annually in banking. This substantial opportunity stems primarily from increased productivity, underlining the transformative potential of these technologies.
Generative AI’s value lies in enhancing and automating human-like processes. Generative AI facilitates data analysis, report generation, and real-time intelligent insights, vital for banking decisions. Programs like Google Bard and ChatGPT, for example, might be useful for finance professionals.
Generative AI automates tasks, freeing finance teams for risk management and strategic planning, boosting productivity and capability. According to BCG, generative AI has the potential to completely change the nature of labor in the finance function. Finance professionals can hence utilize AI tools to boost productivity and spur creativity. Generative AI enhances human abilities, enabling better handling of complex tasks, without replacing workers, as predicted. CFOs need deep understanding of AI’s potential to transform processes, gain competitive advantage.
An S-curve pattern is predicted for the adoption of generative AI in banking, indicating substantial growth potential. The incorporation of generative AI into core operations is anticipated to pick up speed in the upcoming years, even though finance teams are now investigating its potential in fields like text production and research.
Along with streamlining current procedures, this evolution will open the door for new use cases and applications. Generative AI can improve the precision and effectiveness of financial analysis by working with conventional AI forecasting tools, giving banks the ability to make better decisions faster.
Now let’s examine some of the core use cases of Generative AI in banking/financial services:
Generative artificial intelligence (AI) is transforming financial institutions’ operations and customer service, from risk assessment and fraud detection to tailored client experiences.
Below are a few significant AI use cases in banking and other financial services:
Investment Management
Financial advisors use their expertise and technology to manage and invest their clients’ money sensibly. Portfolio managers build and adjust investment portfolios to align with clients’ financial goals and risk preferences.
Asset allocation maximizes returns and minimizes risks through strategic distribution among various asset classes.
Investment managers also offer consulting services, offering conclusions and suggestions derived from economic and market research.
The integration of generative AI into investment management blends technical tools with financial acumen, aiming to optimize client returns while adhering to predefined risk parameters. This approach fosters the accumulation and preservation of wealth through a systematic and well-planned strategy.
Chatbots and Virtual Assistants
Chatbots and virtual assistants have become indispensable in the banking industry, improving client service and engagement.
Generative artificial intelligence is crucial for virtual agents to deliver human-like, contextually relevant responses in fluid and dynamic dialogues.
Generative AI chatbots boost efficiency by handling numerous queries simultaneously with consistent, standardized responses.
Financial firms save money by streamlining customer service operations, reducing the need for extensive human resources. Generative AI lowers costs, enhances customer service, automates tasks, and ensures reliable responses, enhancing overall customer experience.
Fraud Detection and Prevention
Financial industry struggles detecting and preventing fraud due to advanced techniques surpassing traditional rule-based systems’ capabilities.
Financial institutions use AI to simulate and improve fraud detection systems in order to combat this. In the face of this growing threat, generative AI improves fraud detection systems’ ability to adjust to new strategies, increasing their overall accuracy and efficacy.
It is essential for training machine learning models for fraud prediction in addition to helping with system testing and improvement.
Algorithms use fake data in training to reduce false positives/negatives, enhancing fraud detection accuracy. Proactive security measures protect client assets, ensure seamless experiences, and minimize financial losses from fraud.
Personalized Customer Experience
In the banking and other financial sectors, providing individualized customer experiences is crucial since customers are looking for more customized solutions that meet their demands. In order to do this, financial institutions can offer individualized financial advise and design specialized investment portfolios.
One effective instrument for this is generative AI. Generative AI algorithms create recommendations tailored to each customer’s particular situation by evaluating enormous volumes of customer data, including transaction history and financial objectives.
This promotes trust and loyalty. Generally, enhanced retention, loyalty, favorable referrals, and a competitive edge in the market are all consequences of generative AI’s impact on customer engagement and satisfaction levels.
Risk Assessment and Credit Scoring
In the banking industry, risk assessment and credit scoring are crucial. AI brings innovation by producing synthetic data that is useful for model training.
Institutions use synthetic data to depict various risk scenarios, enhancing prediction precision and power. Generative artificial intelligence enhances creditworthiness evaluation, improving loan approval decisions and credit scoring accuracy via customer data analysis.
Furthermore, proactive risk management is made possible by AI, which makes scenario simulation and risk factor analysis easier.
Financial organizations enhance risk management by identifying developing hazards, dependencies, and correlations with synthetic data representing various scenarios.
The system streamlines risk assessment without sacrificing accuracy, optimizing capital allocation and shortening turnaround times through automation.
Cybersecurity and Risk Management
Significant cybersecurity concerns face the banking industry and other financial services due to the sensitive nature of data and high-value transactions. Generative AI plays a crucial role in mitigating these issues by mimicking cyberattacks to evaluate and improve security protocols.
With the use of machine learning algorithms, it enables the real-time identification and mitigation of risks, offering prompt answers to possible breaches. By examining past data and seeing trends, generative AI models forecast and anticipate cybersecurity threats, allowing for proactive risk reduction.
By encrypting critical data, tracking user activity, and detecting unwanted access, this system improves cybersecurity protections. Financial institutions strengthen their security protocols by utilizing generative AI, guaranteeing the safety of client information and upholding confidence in a constantly changing cybersecurity environment.
Loan Underwriting and Mortgage Approval
Efficient mortgage approval and loan underwriting procedures are crucial in banking for optimizing operations and delivering a smooth borrower experience.
Generative AI plays a crucial role in building precise machine learning models, increasing the accuracy of loan underwriting judgments.
Automating document verification and risk assessment in loan underwriting, generative AI saves processing time and increases accuracy, streamlining operations and improving client satisfaction.
This technology expedites approvals, makes customized loan recommendations, resulting in improved loan approval rates, fewer defaults, and increased client satisfaction.
Key Steps for Seamlessly Integrating Generative AI into Your Finance Projects
Embarking on the integration of Generative AI into financial services requires a holistic and strategic approach. By understanding systemic methodologies and partnering with proficient development firms, businesses can effectively harness Generative AI’s transformative potential to foster innovation and achieve their objectives.
Needs Assessment and Goal Definition: Initiate your integration journey with a comprehensive research phase. Conduct a meticulous needs assessment to precisely identify and define the challenges and objectives inherent in your finance projects.
Data Collection and Preparation: Gather financial data comprehensively from diverse sources. Then, meticulously cleanse and preprocess the data to eliminate errors and standardize formats. Augment the dataset with relevant features to enrich its diversity while ensuring regulatory compliance throughout the process to maintain data integrity.
Model Development and Deployment: Utilize the robust dataset to develop and implement Generative AI models tailored specifically to address the identified challenges and meet your defined objectives. Employ suitable algorithms and methodologies to optimize model performance in finance projects.
Testing and Validation: Subject the developed Generative AI models to rigorous testing and validation across a spectrum of finance use cases and scenarios. Identify and rectify any potential shortcomings or discrepancies to ensure the robustness and reliability of the models before deployment.
Integration into Software Workflow: Collaborate closely with software engineers to seamlessly integrate the developed models into existing software workflows within the finance domain. Ensure smooth UI/UX interaction and enhanced operational efficiency through effective integration.
Continuous Monitoring and Optimization: Adopt a proactive approach by implementing continuous monitoring and improvement strategies post-deployment. Track model performance in real-time, conduct data analysis, and implement iterative enhancements to maintain effectiveness and relevance amidst evolving finance trends.
Wrapping Up
Generative AI transforms finance by producing synthetic data, automating processes, and offering invaluable decision-making insights.
It enables smarter investment management, enhanced risk assessment, personalized customer experiences, and better fraud detection beyond real-world data limitations. Advancements in machine learning, coupled with data growth and cost imperatives, drive widespread adoption of generative AI in finance.
At Prakash Software Solutions Pvt. Ltd, we prioritize thought leadership, exploring new avenues to push Generative AI in finance.
Contact us to revolutionize your finance apps with innovative Generative AI solutions!
Happy Reading!!